Evaluators need to be comfortable using AI tools, and they need to understand their strengths and weaknesses. This will help them make informed decisions about how to best use these tools in their evaluations.
AI can help evaluators quickly sift through large amounts of data and identify patterns. This can save evaluators time and allow them to focus on more nuanced aspects of their work.
1. Reliability

Reliability refers to the ability of an AI system to perform as required without failure over time and under given conditions. It also involves the ability to generalize or apply learned information in data and settings outside its original training.
The underlying technology of AI language models such as ChatGPT has shown promise, but additional work is needed to ensure their reliability before clinical integration. Specifically, medical professionals must actively check AI-generated answers and ensure that they are complete and accurate.
2. Responsiveness
Responsiveness refers to the ability to quickly and appropriately address customer questions, concerns, or feedback. It demonstrates a business’s commitment to a positive and lasting relationship with its customers.
In the context of AI, responsiveness includes a number of dimensions and factors. These include a multidimensional AISAQUAL scale that considers efficiency, security, availability, enjoyment, contact, and anthropomorphism. Additionally, constitutional AI can be deployed to moderate socio-cultural biases in generative language models. The deployment of evaluative prototyping is also recommended.
3. Accuracy
Accuracy refers to how close a model’s predictions are to the true values. It’s a metric for assessing an AI program’s performance.
Currently, evaluating the accuracy of AI programs is often done using averages, such as comparing the number of right responses to the number of wrong ones an algorithm gives. But that metric ignores the impact of individual errors.
Creating accurate evaluation metrics and datasets will help to make AI systems more trustworthy. This will include addressing concerns over bias, interpretability and robustness.
4. Efficiency
A key aspect of efficiency is the speed at which AI-based systems complete tasks. This is measured by using evaluation metrics, including classification and regression.
AI technology is also able to automate time-consuming, repetitive tasks, freeing up human evaluators to focus on more high-level activities such as data analysis and strategic decision-making. This improves productivity, enables greater flexibility and facilitates adaptive management strategies.
However, human evaluators are still essential to M&E. They provide domain expertise, contextual understanding and ethical judgment. They must work with AI evaluators to ensure that these strengths are fully leveraged.
5. Flexibility
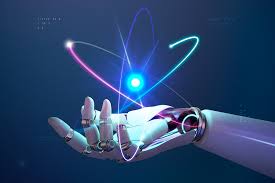
In program evaluation, a variety of methodologies can be used to analyze, collect, and interpret data. AI, particularly NLP and computer vision, can support these processes by automating data collection and facilitating more complex information analysis.
Evaluating AI/ML requires careful attention to detail and patience. However, the process of evaluating an AI model can help organizations unlock its full potential. By aligning technology with needs, ensuring data quality, and evaluating algorithm performance, AI/ML can be a powerful force for innovation and efficiency.
6. Security
The most powerful AI software does fancy data processing but is still software, just like any other type of computer program. Whether you use in-house or external tools, the principles and guardrails established for other types of software must be applied to AI models as well.
For example, you need to ensure that access privileges are tightly controlled and that the infrastructure used by the model is secure. Also, you must be vigilant about detecting false or manipulated training data.
7. Customization
In some cases, the people that does evaluation of the programs have to learn about and become adept with AI tools that do not have a rich history in the field.
For example, new generative AI tools can produce software code based on natural language prompts. They may also be used in IT processes like data entry and fraud detection.
Evaluating these systems can be a bit like peeling back layers of an intricate puzzle. It requires patience and precision, along with a keen eye for detail.
8. Value
AI enables new ways of bringing value to the company and its stakeholders. A sensitivity simulation that compares a traditional business plan without AI with one that incorporates it can show the improved margins and sustainability that come from this new approach.
Karthigan emphasizes that achieving this requires structured effort. Leaders need to help employees understand that their initial reluctance to use AI will be replaced by demand once they see that it is not about replacing them.